Project Leaders
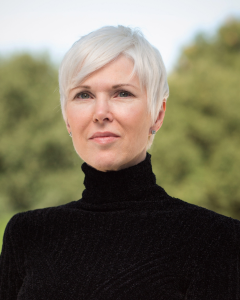
Contact PI
LEANNE WILLIAMS
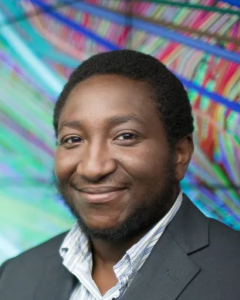
MPI
OLUSOLA A. AJILORE
Professor of Psychiatry
University of Illinois Center for Depression and Resilience (UI CDR)
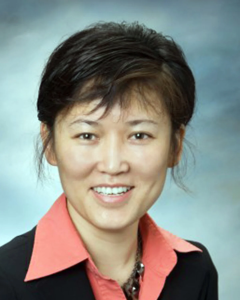
MPI
JUN MA
Professor of Academic Internal Medicine and Geriatrics and Associate Head for Research in the Department of Medicine
University of Illinois Chicago
Project Number
Awardee Organization
STANFORD UNIVERSITY
Program Official
Project Description
Lacking highly accessible, individual-level measurement suited to informing clinical decision-making is a critically unmet need in depression, the leading cause of disability worldwide. Cognitive impairment is a major contributor to disability due to depression. Our team has pioneered the identification of a cognitive phenotype of depression derived from computerized behavioral ‘WebNeuro’ tasks that align with the RDoC cognitive control construct. Behavioral performance on these tasks predicts response outcomes for standard antidepressants, and poor cognitive control is associated with reduced neural circuit activation and poorer quality of life. To optimize these cognitive control behavioral tasks for individual-level predictions, beyond group differences, we propose to (1) develop a personalized clinical cognitive signature that is modelled on individual-level behavioral performance and linked to the neural basis based on functional neuroimaging measures of cognitive control circuit activity, (2) deploy it in combination with a smartphone application to integrate finer-grained, passively sampled behavioral metrics into predictive models, and (3) validate it clinically for prospective assignment of personalized treatment selection. In response to RFA-MH-23-105, our specific aims are as follows:
AIM 1. Optimize a novel clinical cognitive signature for individual-level predictions using unique, large existing multi-modal datasets. To optimize our prior identified cognitive phenotype, we will leverage a large existing dataset of common cognitive data elements and diverse participants with major depressive disorder (MDD) from 21 sites in 5 countries, who were assessed pre-post pharmacotherapy (n=1008), pre-post behavioral therapy (n=108) and following naturalistic trajectories (n=580), and matched healthy participants from the same sites (n=1,386). We hypothesize that an optimized clinical cognitive signature derived from individual-level trial-by-trial performance on WebNeuro, with norm modelling, will predict, beyond clinical record measures, both 1a. naturalistic trajectories of patient-reported outcomes (PROs), including symptom severity, disability and quality of life, over 12 months and 1b. randomized treatment response and remission outcomes; and 1c. will be associated with neural correlates within the cognitive control circuit in a subset of 30% with imaging data.
AIM 2. Prospectively evaluate the clinical cognitive signature in combination with digital metrics for predicting clinical trajectories at scale in a new cohort. We will use our team’s established strategies to recruit a large prospective cohort of 1,200 adults with depression, representative of racially/ethnically and socioeconomically diverse participants. WebNeuro cognitive control tasks will be administered remotely to derive the optimized clinical cognitive signature at weeks 1, 2 and 8. We will incorporate our investigators’ innovative app, BiAffect, to continuously and passively sample digital metrics during this period, complementing WebNeuro with finer-grained individual variations in behavior throughout the day. We will evaluate whether individuals with the signature have different outcome patterns, such as longer episodes, slower improvements in symptom severity and worsening of disability and quality of life, than individuals without the signature. The primary PROs, symptom severity, disability and quality of life will be assessed at weeks 1, 2 and 8 and again at 6, 9 and 12 months. We hypothesize that the optimized signature will 2a. show stability over 8 weeks and 2b. predict both concurrent (over 8 weeks) and longitudinal (over 12 months) PROs. The findings will establish generalizability of the signature to a new, diverse sample. Further, we hypothesize that 2c. individual level BiAffect metrics will complement WebNeuro-derived signature to enhance the precision with which the PROs can be predicted.
AIM 3. Prospectively validate the clinical cognitive signature for treatment outcomes in a clinical trial.
We will conduct a parallel-group, double-blind randomized trial at Stanford Bay Area and Chicago sites, identifying 160 participants with a prominent clinical cognitive signature (C+) and relative absence of the signature (C-). We will enrich for C+, the signature of interest, at a 2:1 ratio. Participants will be randomly assigned to receive guanfacine (shown to ameliorate cognitive control deficits in our preliminary data) plus sertraline or placebo plus sertraline. The primary outcome is treatment remission. We hypothesize that 3a. signature status (C+ v C-) will predict treatment outcome, beyond clinical record measures, as a function of treatment arm; 3b. individual level BiAffect metrics will complement the signature status (C+ v C-) to further enhance the precision of prediction of treatment outcomes, as a function of treatment arm; and 3c. C+ individuals will be distinguished from C- by reduced activity within the cognitive control circuit and circuit activity measures will further enhance the precision of prediction of treatment outcomes as a function of treatment arm.
Significance, Deliverables and Synergy. The proposed stellar research team has a track record of collaboration, synergizing multidisciplinary expertise in the successful establishment, coordination, and management of large-scale research projects, clinical practice in mental health, behavioral assessment, phenotype development and validation, statistical modelling and biostatistics, participant recruitment and retention, ethical considerations and lived experience. The team spans diverse backgrounds, including underrepresented groups, life experiences and career stages. Deliverables will include a behavioral assessment tool suited to implementation at scale, ready for near-term translation into routine clinical practice. It will offer a clinical cognitive signature that can inform prognostic and tailored treatment decisions. The project will generate a unique FAIR-compliant dataset and data structures that enable future scaling using machine learning and AI.