Project Leaders
Project Number
1U24MH136069-01
Awardee Organization
YALE UNIVERSITY
Program Official
MICHELE FERRANTE
Project Description
Mental health research faces significant challenges, including the heterogeneity of diagnostic groups and the lack of precise characterization of individual patients, hindering effective clinical decision-making. However, data-driven approaches, such as machine learning and computational analyses, have emerged as crucial tools to address these challenges. By integrating data from behavioral assessments, clinical records, and biological markers, these approaches can generate more precise and objective clinical phenotypes, leading to improved diagnostic accuracy, personalized treatment selection, mechanistic insights, and enhanced monitoring and prognosis. The use of data-driven approaches holds immense importance in revolutionizing mental health research, enabling tailored interventions, and advancing our understanding and management of mental disorders. By integrating diverse data sources and leveraging advanced computational techniques, the Individually Measured Phenotypes to Advance Computational Translation in Mental Health (IMPACT-MH) initiative was formed, with the goal to harness the power of big data to address the complexity and heterogeneity of mental disorders, ultimately improving patient care and outcomes.
Mental disorders exhibit complex characteristics, making it difficult to represent, collect, and analyze heterogeneous data effectively. One major challenge is the absence of a unified representation for mental health data. While the Research Domain Criteria framework (RDoC) aims to provide a systematic framework, a formal representation using existing biomedical standards, such as ontologies, is still lacking. Developing such standards is crucial to generate computational phenotypes. Additionally, once data standards and normalization methods are established, disseminating them to researchers is essential for promoting the generation of interoperable and reusable data. Moreover, ensuring the generalizability of phenotyping algorithms beyond their original development institute and minimizing bias associated with potential errors are critical factors for enabling broad applications of such algorithms in mental health research. Addressing these challenges is pivotal for advancing computational phenotyping in mental health and facilitating its broader utilization. To tackle these challenges, three cores were established with three Aims:
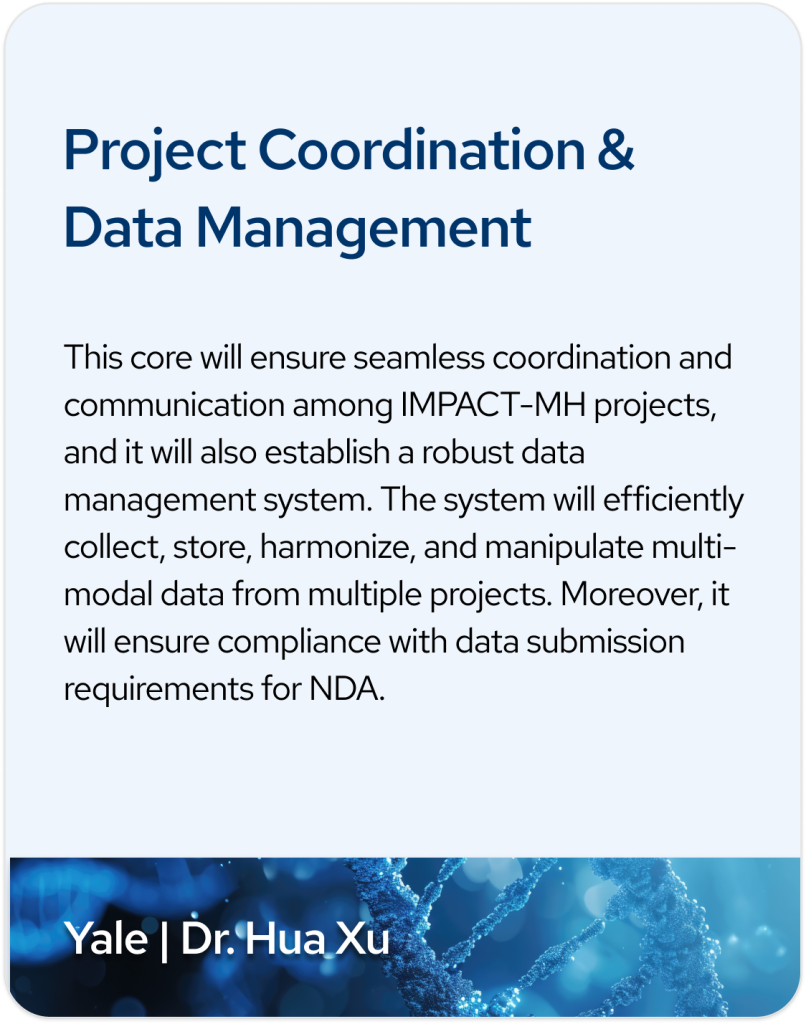
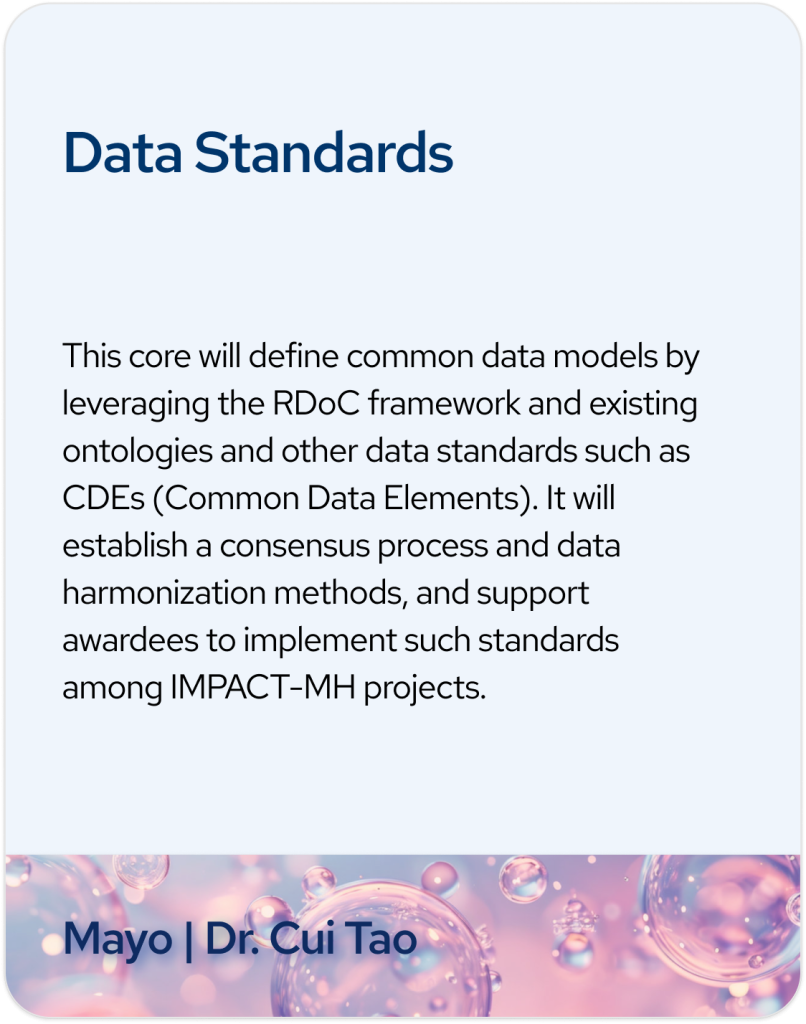
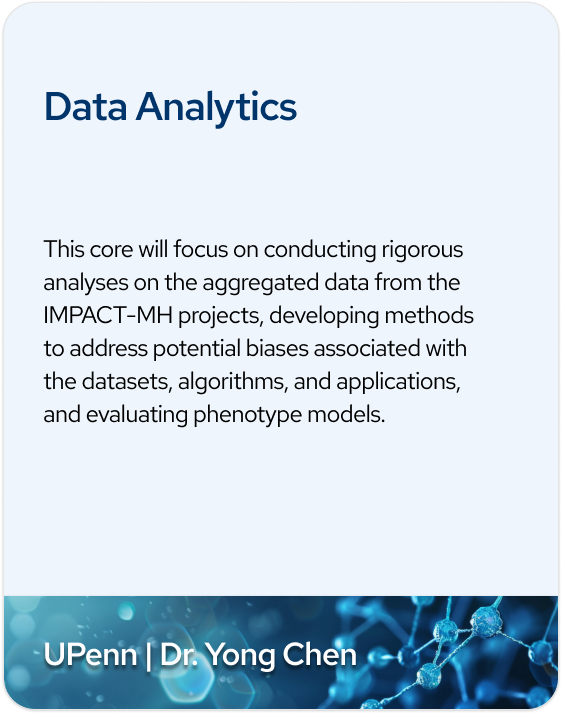