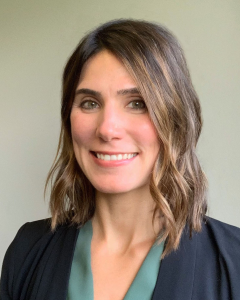
Contact PI
ADRIANE M. SOEHNER
Associate Professor of Psychiatry
University of Pittsburgh
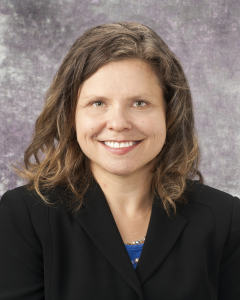
MPI
MARIA JALBRZIKOWSKI
Assistant Professor of Psychology in the Department of Psychiatry
Boston Children’s Hospital / Harvard Medical School
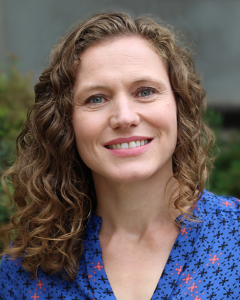
MPI
MEREDITH JOANNE LOTZ WALLACE
Associate Professor of Psychiatry
University of Pittsburgh
Project Number
1U01MH136020-01
Awardee Organization
UNIVERSITY OF PITTSBURGH AT PITTSBURGH
Program Official
ASHLEY SMITH
Project Description
Peri-adolescence (ages 10-13) heralds an alarming escalation in pediatric mental health (MH) risk, making this transitionary time between childhood and adolescence a crucial window for early detection and intervention. Predictive models built on electronic health record (EHR) data are emerging as practical tools to automate MH risk stratification in pediatric primary care (PPC); however, to date these models have only modest predictive value. Common, causal, and modifiable transdiagnostic MH risk factors are needed to improve on EHR models and pave the way for deployment of preventive care during peri-adolescence. Sleep is one such risk factor: more than 50% of peri-adolescents experience unhealthy sleep patterns, and sleep problems during peri-adolescence prospectively predict negative MH outcomes more strongly than at other times in development. Moreover, youth from minoritized racial and ethnic backgrounds are even more severely impacted by poor sleep health than non- minoritized youth. However, several barriers have impeded the incorporation of sleep into PPC screening and risk stratification tools: (1) the heterogeneity of sleep characteristics hinders the differentiation of normative versus at-risk sleep patterns; (2) the multidimensionality and multimodality of sleep data have led to inconsistencies regarding which particular features and modalities are needed for screening; and (3) until very recently, it has been impossible to capture crucial behavioral and physiological measures of habitual sleep in sufficiently large samples. To address these challenges, we propose the Pediatric Precision Sleep Network: a multi-site observational study of unprecedented scope and scale, focused on establishing sleep signatures that predict transdiagnostic MH problems among youth in PPC.
Across three sites, we will recruit a diverse sample of 1,200 youth (10-13yr) with a range of sleep disturbances from PPC settings. We will develop and validate multidimensional and multimodal sleep signatures (Aim 1) and practical and scalable transdiagnostic MH risk stratification algorithms (Aim 2). Aims 1-2 will employ a sequential, multimodal machine learning workflow to establish the extent to which each data modality can incrementally inform signatures and identify youth at greatest transdiagnostic MH risk. These findings will inform screening, risk stratification, and future developmentally-appropriate effectiveness and/or experimental therapeutics trials. In Aim 3, we will coordinate with the IMPACT-MH Data Coordinating Center to efficiently recruit participants, collect data, hone and share innovative data processing pipelines, and securely transfer data. We are committed to bringing sleep and MH data to our broader scientific community, along with the accompanying tools and resources needed to process sleep data efficiently.
Our long-term goal is to inform clinical decision-making algorithms for PPC that leverage sleep to improve MH outcomes and reduce MH disparities at a pivotal developmental window.